Statistical Shape Modeling
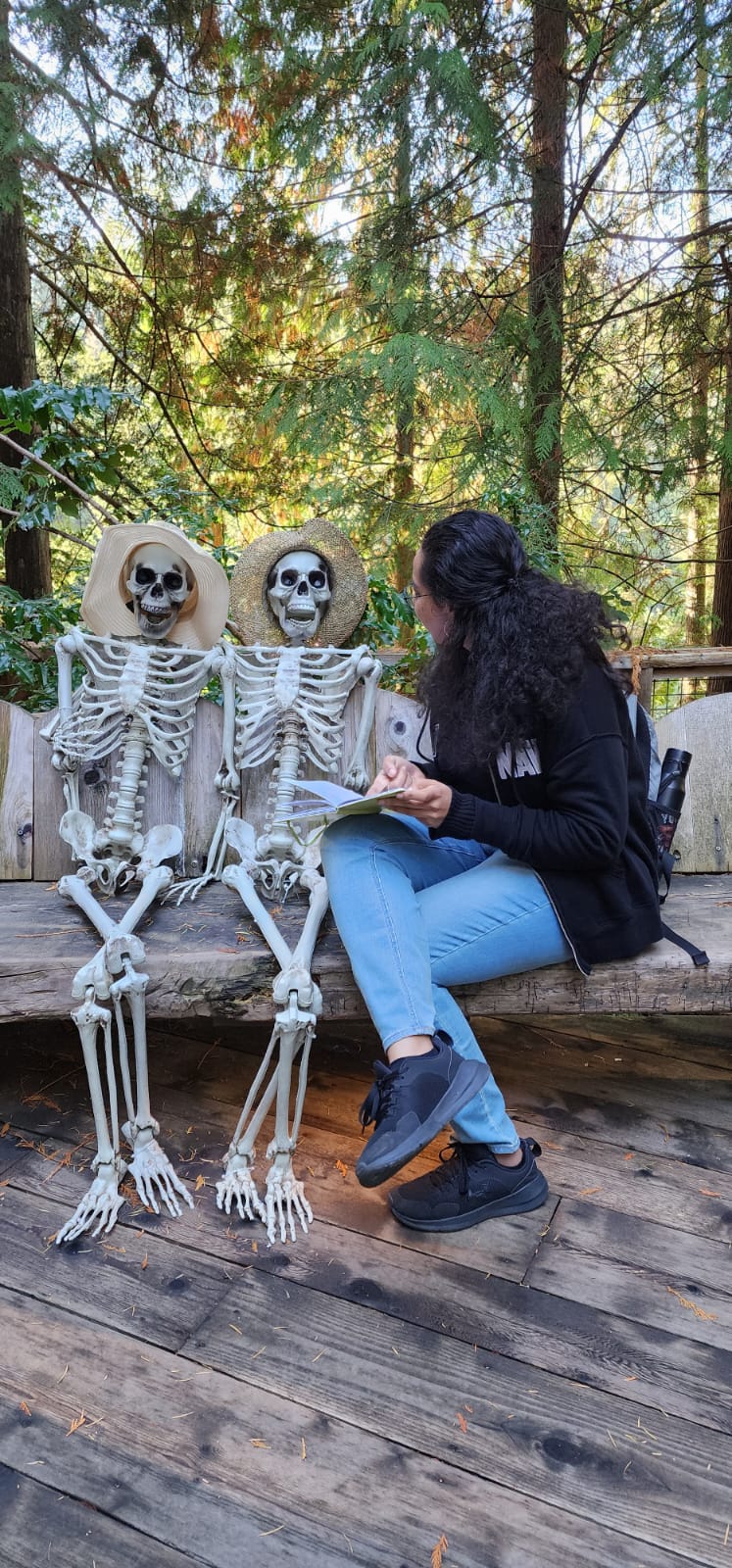
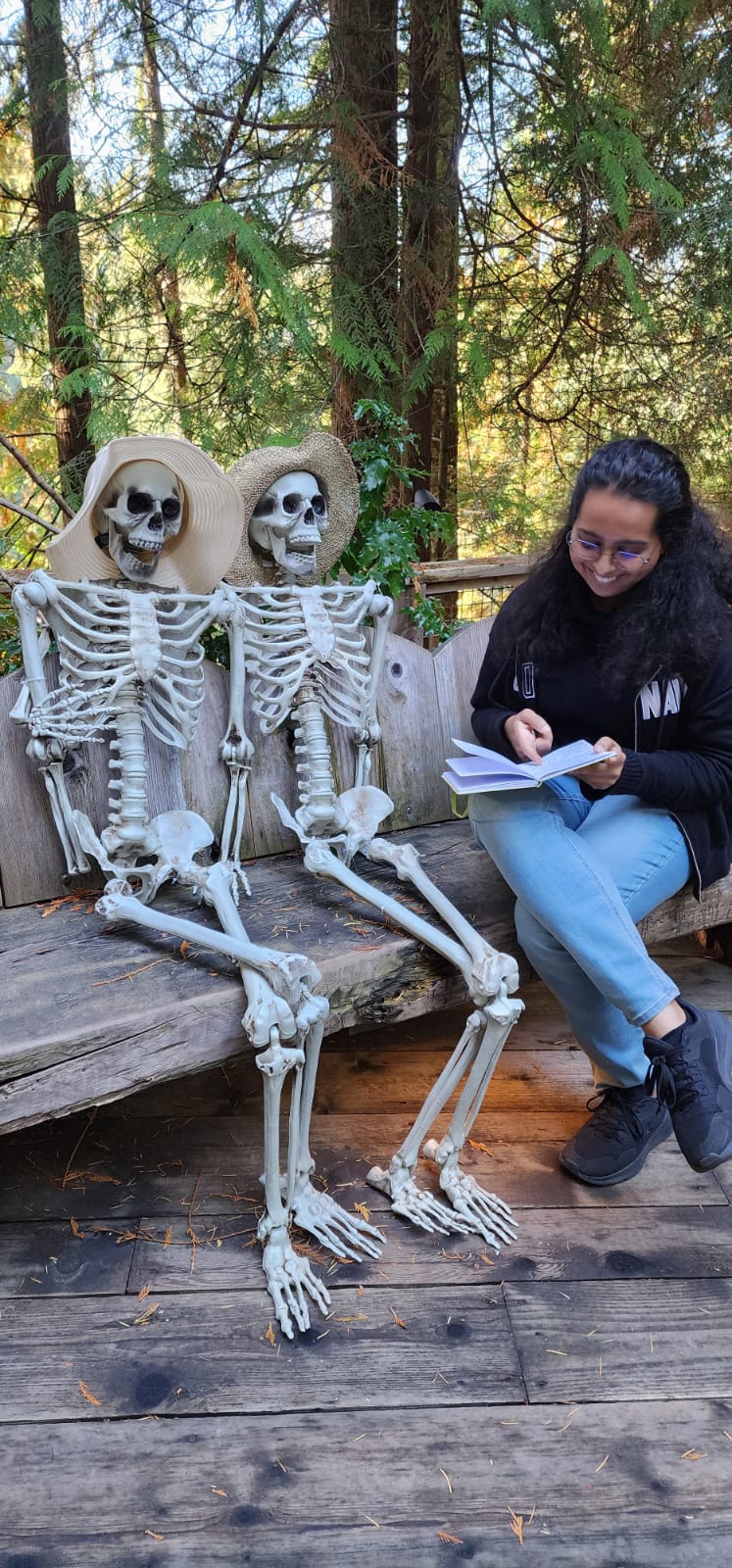
Statistical shape modeling (SSM) is essential for anatomy evaluation in medical image analysis and computational anatomy. SSM facilitates the discovery of quantitative morphological shape descriptors from medical imaging data such as MRI and CT scans. These descriptors comprehensively describe anatomical variations within the context of the population.
My research addresses limitations in shape modeling pipelines, introducing innovative computational methodologies to enhance model robustness and applicability across diverse clinical scenarios, ultimately aiming to advance personalized diagnostic and treatment approaches.
Objective 1: Benchmarking and effective utilization of SSM tools for clinical application
Current evaluations predominantly focus on non-clinical domains, leaving a gap in understanding the applicability of SSM techniques in real-world clinical scenarios. We aim to conduct comprehensive evaluation and validation studies to assess the precision and reliability of SSM tools for clinical tasks such as landmark/measurement estimation and lesion screening across multiple datasets. Additionally, I aim to demonstrate the effectiveness of SSM in identifying and quantifying morphological changes indicative of pathology. My work includes detailed benchmarking studies and case studies in cardiology to showcase the potential of SSM in clinical applications.
Objective 2: SSM tools for complex anatomies with shared boundaries
The unique shape changes of the complex anatomical structures consisting of multiple organs with a shared boundary are not captured when the organs are modeled individually. Therefore, this work aims to develop (a) simple and effective shape modeling tools for extracting shared boundary surfaces and (b) a correspondence-based optimization scheme to parameterize multi-organ anatomies and their shared surfaces consistently. The proposed method enables a better understanding of complex anatomical structures and facilitates the detection of subtle pathological changes within shared boundaries.
Objective 3: Enhancing deep learning methods for SSM from surface meshes
Substantial non-linear variability in human anatomy often makes the traditional shape modeling process challenging. Deep learning techniques have the potential to learn complex nonlinear representations of shapes and generate statistical shape models more faithful to the underlying population-level variability. This work aims to predict correspondences from meshes in an unsupervised manner. This approach seeks to overcome the limitations associated with linearity assumption and computationally intensive inference pipelines.

Objective 4: Shape priors for SSM from unsegmented images
Existing deep learning methods for estimating correspondences from CT/MRI images rely heavily on established PDMs for training, limiting their applicability and generalization. Moreover, volumetric images could contain misleading information, effectively regularizing the correspondence estimation process becomes critical for precise feature extraction. This work proposes an approach to predict correspondences directly from raw images without relying on pre-optimized PDMs, leveraging shape prior built from surface representations like meshes, point clouds, or segmentations to guide the image-driven SSM task to learn predictive features.
SCorP: Statistics-Informed Dense Correspondence Prediction Directly from Unsegmented Medical Images
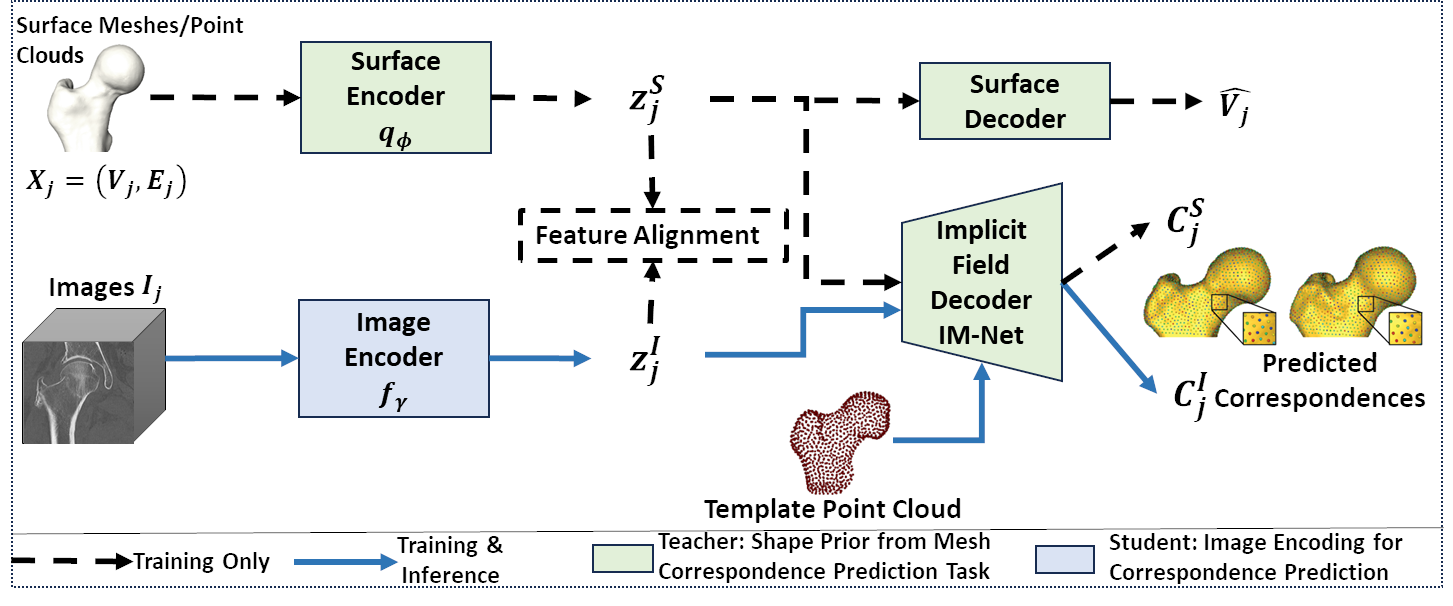
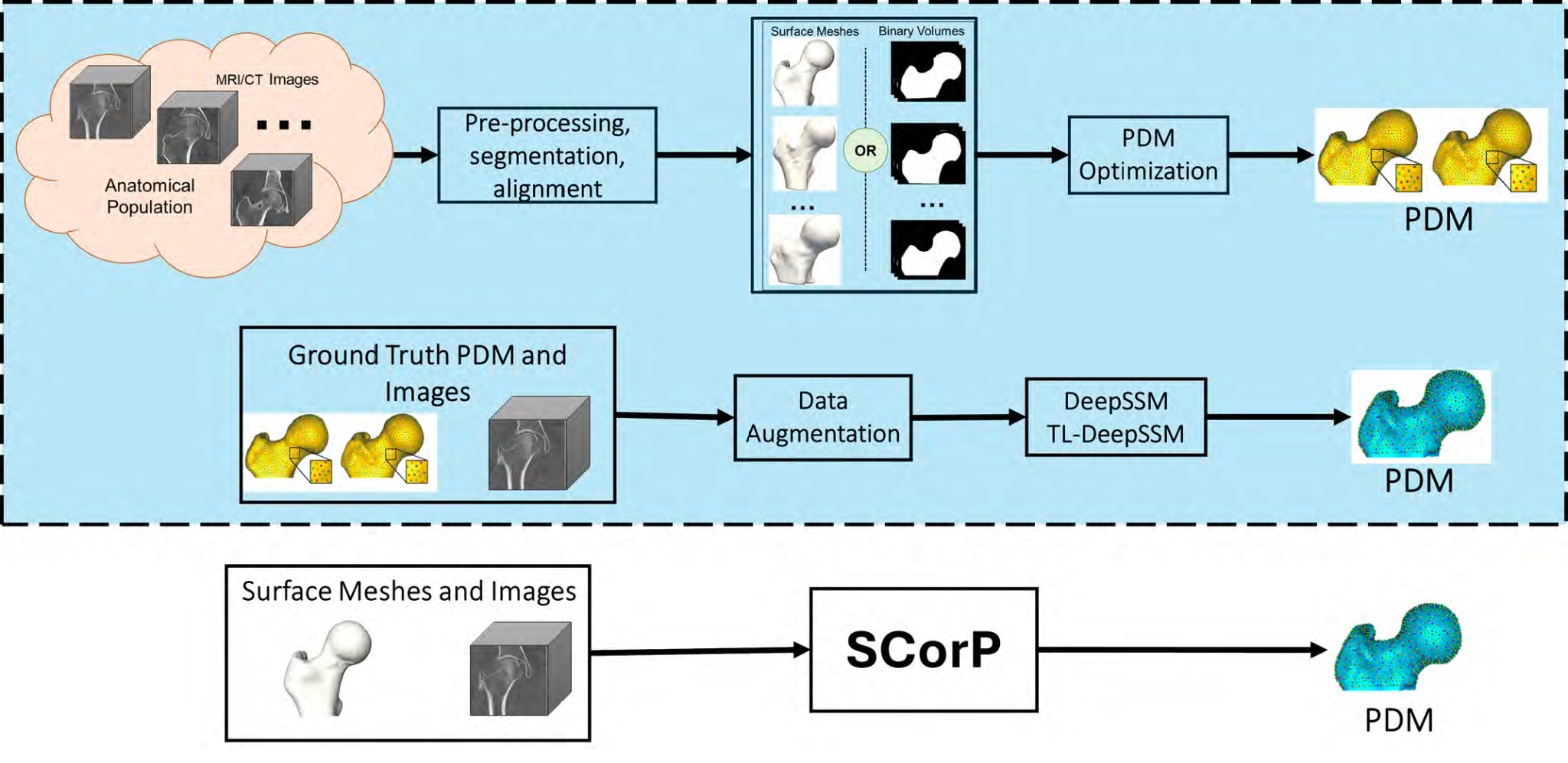