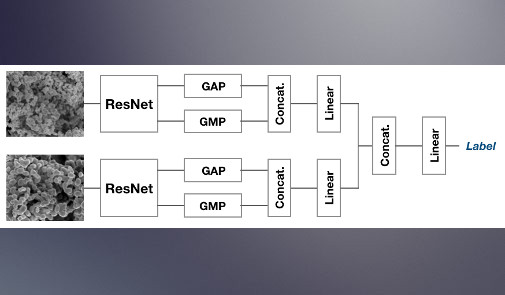
Nuclear forensic analysis via deep learning
Nuclear forensics aims to investigate the origin and history of nuclear or radioactive materials via analytical techniques. Deep learning applied to electron microscopy images can automatically and accurately identify subtle differences in microstructure from different samples. It provides insights into synthesis conditions and processing routes of uranium ore concentrates. We applied, compared and fine-tuned via transfer learning state-of-the-art convolutional neural networks, performing numerous image classification and regression tasks. In addition to applying standard neural network architectures (ResNet, DenseNet), we implemented parallel networks to study multi-magnification acquisitions, and quantified model uncertainty during inference.