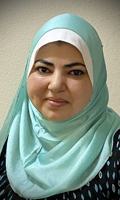
Dr. Shireen Elhabian - Associate Professor of Computer Science
WEB 3608fax (801) 585-6513
Personal Home Page
Background
Dr. Elhabian received her Ph.D. in Electrical and Computer Engineering from the University of Louisville in 2012, in the field of statistical and mathematical modeling of photographic images, with a focus on computer vision and medical image analysis. She was a postdoctoral fellow from 2013 to 2016, and a research scientist from 2016 to 2017, both at the SCI Institute, University of Utah. Before joining the tenure-line faculty, she was a research computer scientist within the SCI Institute and a research assistant professor with the School of Computing from 2017 to 2022. She is also an adjunct faculty in the Department of Electrical & Computer Engineering at the University of Utah.
Current Responsibilities
Dr. Elhabian has established her research program around biomedical problems that entail collaborating with scientists and domain experts of different disciplines and backgrounds to conduct interdisciplinary research projects. Her work spans foundational and translational research at the intersection of image analysis and statistical machine learning and a focus on clinical and biomedical applications.
Dr. Elhabian’s vision is that deployable image analysis systems empowered by machine learning can transform the way biomedical researchers and clinicians interpret imaging data in an objective, thorough, efficient, and reproducible manner, thereby maximizing the benefit-to-cost of imaging technologies and enabling early diagnosis and patient-specific treatment and prognosis. Her long-term goal is to accelerate the adoption and increase the clinical utility of machine-learning-based image analysis systems that mitigate critical bottlenecks in attaining an expert-level understanding of the complexities of imaging data and have a broad impact in a range of clinical and biomedical research disciplines. To attain this goal, Dr. Elhabian has been establishing foundational methods to solve inverse problems in image analysis and quantitatively interpret imaging data using minimal expert supervision, and translating these methods to application domains through robust, flexible, and usable open-source software packages.
Dr. Elhabian’s vision is that deployable image analysis systems empowered by machine learning can transform the way biomedical researchers and clinicians interpret imaging data in an objective, thorough, efficient, and reproducible manner, thereby maximizing the benefit-to-cost of imaging technologies and enabling early diagnosis and patient-specific treatment and prognosis. Her long-term goal is to accelerate the adoption and increase the clinical utility of machine-learning-based image analysis systems that mitigate critical bottlenecks in attaining an expert-level understanding of the complexities of imaging data and have a broad impact in a range of clinical and biomedical research disciplines. To attain this goal, Dr. Elhabian has been establishing foundational methods to solve inverse problems in image analysis and quantitatively interpret imaging data using minimal expert supervision, and translating these methods to application domains through robust, flexible, and usable open-source software packages.
Research Interests
- Medical image analysis
- Machine learning and deep learning
- Probablistic and mathematical modeling
- Shape modeling and analysis
- Image processing
- Computer vision