SCI Publications
2007
C.R. Butson, S.E. Cooper, J.M. Henderson, C.C. McIntyre.
Patient-specific analysis of the volume of tissue activated during deep brain stimulation, In NeuroImage, Vol. 34, No. 2, pp. 661--670. January, 2007.
ISSN: 1053-8119
DOI: 10.1016/j.neuroimage.2006.09.034
PubMed ID: 17113789
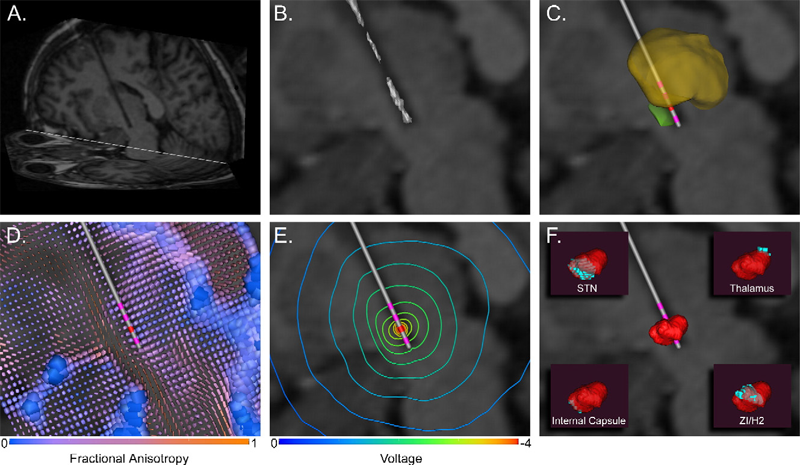
Keywords: Deep Brain Stimulation, Finite Element Analysis, Humans, Imaging, Three-Dimensional, Magnetic Resonance Imaging, Models, Neurological, Parkinson Disease, Parkinson Disease: therapy, Pyramidal Tracts, Pyramidal Tracts: physiology, Subthalamic Nucleus, Subthalamic Nucleus: physiology
C.J. Cascio, G. Gerig, J. Piven.
Diffusion Tensor Imaging: Application to the Study of the Developing Brain, In J Am Acad Child Adolesc Psychiatry, Vol. 46, No. 2, pp. 213--223. February, 2007.
P.T. Fletcher, S. Joshi.
Riemannian Geometry for the Statistical Analysis of Diffusion Tensor Data, In Signal Processing, Vol. 87, No. 2, pp. 250--262. February, 2007.
P.T. Fletcher, R. Tao, W.-K. Jeong, R.T. Whitaker.
A Volumetric Approach to Quantifying Region-to-Region White Matter Connectivity in Diffusion Tensor MRI, In Information Processing in Medical Imaging, Vol. 4584/2007, pp. 346--358. 2007.
C. Goodlett, P.T. Fletcher, W. Lin, G. Gerig.
Quantification of Measurement Error in DTI: Theoretical Predictions and Validation, In Proceedings of The 10th International Conference on Medical Image Computing and Computer Assisted Intervention (MICCAI 2007), Lecture Notes in Computer Science, Vol. 4792, pp. 10--17. November, 2007.
PubMed ID: 18051038
C.B. Goodlett, P.T. Fletcher, W. Lin, G. Gerig.
Noise-Induced Bias in Low-Direction Diffusion Tensor MRI: Replication of Monte-Carlo Simulation with In-Vivo Scans, In Proceedings of ISMRM 2007, 2007.
W.-K. Jeong, P.T. Fletcher, R. Tao, R.T. Whitaker.
Interactive Visualization of Volumetric White Matter Connectivity in DT-MRI Using a Parallel-Hardware Hamilton-Jacobi Solver, In IEEE Transactions on Visualization and Computer Graphics, Vol. 13, No. 6, pp. 1480--1487. 2007.
PubMed ID: 17968100
G. Kindlmann, D.B. Ennis, R.T. Whitaker, C.-F. Westin.
Diffusion Tensor Analysis With Invariant Gradients and Rotation Tangents, In IEEE Transactions on Medical Imaging, Vol. 26, No. 11, pp. 1483--1499. 2007.
F. Zhang, C. Goodlett, E. Hancock, G. Gerig.
Probabilistic White Matter Fiber Tracking using Particle Filtering, In Proceedings of The 10th International Conference on Medical Image Computing and Computer Assisted Intervention (MICCAI 2007), Lecture Notes in Computer Science, Vol. 4791, pp. 144--151. November, 2007.
2006
S. Basu, P.T. Fletcher, R.T. Whitaker.
Rician Noise Removal in Diffusion Tensor MRI, In Medical Image Computing and Computer Assisted Intervention (MICCAI), Vol. LNCS 4190, pp. 117--125. October, 2006.
C.J. Cascio, G. Gerig, J. Piven.
Diffusion Tensor Imaging: Application to the Study of the Developing Brain, In Journal of the American Academy of Child and Adolescent Psychiatry JAACAP, August, 2006.
I. Corouge, P.T. Fletcher, S. Joshi, S. Gouttard, G. Gerig.
Fiber Tract-Oriented Statistics for Quantitative Diffusion Tensor MRI Analysis, In Medical Image Analysis, Vol. 10, No. 5, pp. 786--798. 2006.
G. Kindlmann, X. Tricoche, C.-F. Westin.
Anisotropy Creases Delineate White Matter Structure in Diffusion Tensor MRI, In Proceedings of the Ninth International Conference on Medical Image Computing and Computer-Assisted Intervention (MICCAI'06), Copenhagen, Denmark, Springer Lecture Notes in Computer Science 4190, pp. 126--133. October, 2006.
T. Schultz, B. Burgeth, J. Weickert.
Flexible Segmentation and Smoothing of DT-MRI Fields Through a Customizable Structure Tensor, In Advances in Visual Computing, Lecture Notes in Computer Science, Vol. 4291, Edited by G. Bebis and R. Boyle and B. Parvin and D. Koracin and P. Remagnino and A. V. Nefian and M. Gopi and V. Pascucci and J. Zara and J. Molineros and H. Theisel and T. Malzbender, Springer, pp. 455--464. 2006.
2005
I. Corouge, P.T. Fletcher, S. Joshi, J.H. Gilmore, G. Gerig.
Fiber Tract-Oriented Statistics for Quantitative Diffusion Tensor MRI Analysis, In Med Image Comput Comput Assist Interv Int Conf Med Image Comput Comput Assist Interv, Vol. 8 (Pt. 1), pp. 131--139. 2005.
S. Zhang, D.H. Laidlaw, G. Kindlmann.
Diffusion Tensor MRI Visualization, In The Visualization Handbook, Edited by C.D. Hansen and C.R. Johnson, Elsevier, pp. 327--340. 2005.
ISBN: 0-12-387582-X
2004
A.B. Cheryauka, J.N. Lee, A.A. Samsonov, M. Defrise, G.T. Gullberg.
MRI Diffusion Tensor Reconstruction with PROPELLER Data Acquisition, In J. Mag. Res. Imag., Vol. 22, No. 2, pp. 139--148. 2004.
G Kindlmann.
Superquadric Tensor Glyphs, In Proceeding of The Joint Eurographics - IEEE TCVG Symposium on Visualization 2004, pp. 147--154. May, 2004.
G. Kindlmann.
Visualization and Analysis of Diffusion Tensor Fields, Technical Report, No. UUCS-04-014, Note: PhD Thesis, School of Computing, University of Utah, 2004.
A.A. Samsonov, C.R. Johnson..
Removal of Intensity Bias in Magnitude Spin-Echo MRI Images by Nonlinear Diffusion Filtering, In Proceedings of SPIE Medical Imaging 2004, San Diego, CA, Vol. 5370, pp. 743--752. 2004.
Page 3 of 4