SCI Publications
2010
B.C. Davis, P.T. Fletcher, E. Bullitt, S. Joshi.
Population Shape Regression from Random Design Data, In International Journal of Computer Vision, Vol. 90, No. 1, Note: Marr Prize Special Issue, pp. 255--266. October, 2010.
DOI: 10.1109/ICCV.2007.4408977
N.J. Drury, B.J. Ellis, J.A. Weiss, P.J. McMahon, R.E. Debski.
The impact of glenoid labrum thickness and modulus on labrum and glenohumeral capsule function, In Journal of Biomechanical Engineering, Vol. 132, No. 12, Note: Awarded 2010 Skalak Best Paper!, pp. 121003--121010. 2010.
DOI: 10.1115/1.4002622
S. Durrleman, X. Pennec, A. Trouvé, N. Ayache, J. Braga.
Comparison of the endocast growth of chimpanzees and bonobos via temporal regression and spatiotemporal registration, In Proceedings of the MICCAI Workshop on Spatio-Temporal Image Analysis for Longitudinal and Time-Series Image Data, Beijing, China, pp. (in press). September, 2010.
S. Durrleman, P. Fillard, X. Pennec, A. Trouvé, N. Ayache.
Registration, Atlas Estimation and Variability Analysis of White Matter Fiber Bundles Modeled as Currents, In NeuroImage, Vol. 55, No. 3, pp. 1073--1090. 2010.
DOI: 10.1016/j.neuroimage.2010.11.056
M. El-Sayed, R.G. Steen, M.D. Poe, T.C. Bethea, G. Gerig, J. Lieberman, L. Sikich.
Deficits in gray matter volume in psychotic youth with schizophrenia-spectrum disorders are not evident in psychotic youth with mood disorders, In J Psychiatry Neurosci, July, 2010.
M. El-Sayed, R.G. Steen, M.D. Poe, T.C. Bethea, G. Gerig, J. Lieberman, L. Sikich.
Brain volumes in psychotic youth with schizophrenia and mood disorders, In Journal of Psychiatry and Neuroscience, Vol. 35, No. 4, pp. 229--236. July, 2010.
PubMed ID: 20569649
T. Etiene, L.G. Nonato, C.E. Scheidegger, J. Tierny, T.J. Peters, V. Pascucci, R.M. Kirby, C.T. Silva.
Topology Verification for Isosurface Extraction, SCI Technical Report, No. UUSCI-2010-003, SCI Institute, University of Utah, 2010.
P.T. Fletcher, R.T. Whitaker, R. Tao, M.B. DuBray, A. Froehlich, C. Ravichandran, A.L. Alexander, E.D. Bigler, N. Lange, J.E. Lainhart.
Microstructural connectivity of the arcuate fasciculus in adolescents with high-functioning autism, In NeuroImage, Vol. 51, No. 3, Note: Epub Feb 2 2010, pp. 1117--1125. July, 2010.
PubMed ID: 20132894
T. Fogal, J. Krüger.
Tuvok, an Architecture for Large Scale Volume Rendering, In Proceedings of the 15th International Workshop on Vision, Modeling, and Visualization, pp. 139--146. November, 2010.
DOI: 10.2312/PE/VMV/VMV10/139-146
T. Fogal, H. Childs, S. Shankar, J. Krüger, R.D. Bergeron, P. Hatcher.
Large Data Visualization on Distributed Memory Multi-GPU Clusters, In Proceedings of High Performance Graphics 2010, pp. 57--66. 2010.
S.E. Geneser, J.D. Hinkle, R.M. Kirby, Brian Wang, B. Salter, S. Joshi.
Quantifying Variability in Radiation Dose Due to Respiratory-Induced Tumor Motion, In Medical Image Analysis, Vol. 15, No. 4, pp. 640--649. 2010.
DOI: 10.1016/j.media.2010.07.003
S. Gerber, T. Tasdizen, P.T. Fletcher, S. Joshi, R.T. Whitaker, the Alzheimers Disease Neuroimaging Initiative (ADNI).
Manifold modeling for brain population analysis, In Medical Image Analysis, Special Issue on the 12th International Conference on Medical Image Computing and Computer Assisted Intervention (MICCAI) 2009, Vol. 14, No. 5, Note: Awarded MICCAI 2010, Best of the Journal Issue Award, pp. 643--653. 2010.
ISSN: 1361-8415
DOI: 10.1016/j.media.2010.05.008
PubMed ID: 20579930
S. Gerber, P.-T. Bremer, V. Pascucci, R.T. Whitaker.
Visual Exploration of High Dimensional Scalar Functions, In IEEE Transactions on Visualization and Computer Graphics, IEEE Transactions on Visualization and Computer Graphics, Vol. 16, No. 6, IEEE, pp. 1271--1280. Nov, 2010.
DOI: 10.1109/TVCG.2010.213
PubMed ID: 20975167
PubMed Central ID: PMC3099238
J.H. Gilmore, C. Kang, D.D. Evans, H.M. Wolfe, M.D. Smith, J.A. Lieberman, W. Lin, R.M. Hamer, M. Styner, G. Gerig.
Prenatal and Neonatal Brain Structure and White Matter Maturation in Children at High Risk for Schizophrenia, In American Journal of Psychiatry, Vol. 167, No. 9, Note: Epub 2010 Jun 1, pp. 1083--1091. September, 2010.
PubMed ID: 20516153
J.H. Gilmore, J.E. Schmitt, R.C. Knickmeyer, J.K. Smith, W. Lin, M. Styner, G. Gerig, M.C. Neale.
Genetic and environmental contributions to neonatal brain structure: A twin study, In Human Brain Mapping, Vol. 31, No. 8, Note: ePub 8 Jan 2010, pp. 1174--1182. 2010.
PubMed ID: 20063301
K. Gorczowski, M. Styner, J.Y. Jeong, J.S. Marron, J. Piven, H.C. Hazlett, S.M. Pizer, G. Gerig.
Multi-object analysis of volume, pose, and shape using statistical discrimination, In IEEE Trans Pattern Anal Mach Intell., Vol. 32, No. 4, pp. 652--661. April, 2010.
DOI: 10.1109/TPAMI.2009.92
PubMed ID: 20224121
M. Grabherr, P. Russell, M.D. Meyer, E. Mauceli, J. Alföldi, F. Di Palma, K. Lindblad-Toh.
Genome-wide synteny through highly sensitive sequence alignment: Satsuma, In Bioinformatics, Vol. 26, No. 9, pp. 1145--1151. 2010.
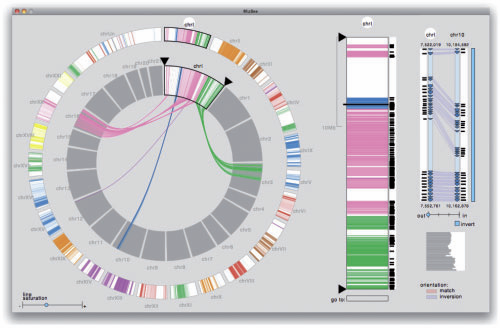
Motivation: Comparative genomics heavily relies on alignments of large and often complex DNA sequences. From an engineering perspective, the problem here is to provide maximum sensitivity (to find all there is to find), specificity (to only find real homology) and speed (to accommodate the billions of base pairs of vertebrate genomes).
Results: Satsuma addresses all three issues through novel strategies: (i) cross-correlation, implemented via fast Fourier transform; (ii) a match scoring scheme that eliminates almost all false hits; and (iii) an asynchronous 'battleship'-like search that allows for aligning two entire fish genomes (470 and 217 Mb) in 120 CPU hours using 15 processors on a single machine.
Availability: Satsuma is part of the Spines software package, implemented in C++ on Linux. The latest version of Spines can be freely downloaded under the LGPL license from http://www.broadinstitute.org/science/programs/genome-biology/spines/ Contact: grabherr@broadinstitute.org
X. Guo, J. Li, D. Xiu, A. Alexeenko.
Uncertainty Quantification Models for Microscale Squeeze-Film Damping, In International Journal for Numerical Methods in Engineering, Vol. 84, No. 10, pp. 1257--1272. 2010.
DOI: 10.1002/nme.2952
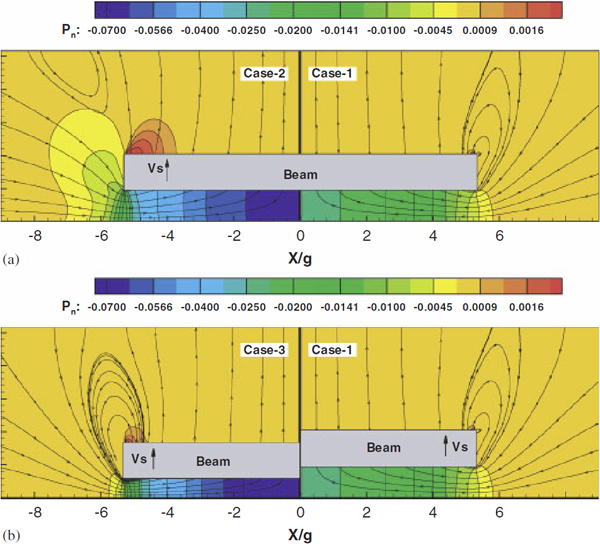
Keywords: uncertainty quantification, squeeze-film damping, gPC expansion, rarefied flow
L. Ha, M.W. Prastawa, G. Gerig, J.H. Gilmore, C.T. Silva, S. Joshi.
Image Registration Driven by Combined Probabilistic and Geometric Descriptors, In Med Image Comput Comput Assist Interv., Vol. 13, No. 2, pp. 602--609. 2010.
PubMed ID: 20879365
L.K. Ha, J. Krüger, S. Joshi, C.T. Silva.
Multi-scale Unbiased Diffeomorphic Atlas Construction on Multi-GPUs, In GPU Computing Gems, Vol. 1, 2010.
In this chapter, we present a high performance multi-scale 3D image processing framework to exploit the parallel processing power of multiple graphic processing units (Multi-GPUs) for medical image analysis. We developed GPU algorithms and data structures that can be applied to a wide range of 3D image processing applications and efficiently exploit the computational power and massive bandwidth offered by modern GPUs. Our framework helps scientists solve computationally intensive problems which previously required super computing power. To demonstrate the effectiveness of our framework and to compare to existing techniques, we focus our discussions on atlas construction - the application of understanding the development of the brain and the progression of brain diseases.
Page 66 of 144