SCI Publications
2002
Y. Serinagaoglu, R.S. MacLeod, B. Yilmaz, D.H. Brooks.
Multielectrode Venous Catheter Mapping as a High Quality Constraint for Electrocardiographic Inverse Solution, In J. Electrocardiol., Vol. 35 (supplement), 2002.
J. Simpson, A.R. Sanderson, E. Luke, K. Balling, R. Coffey.
Collaborative Remote Visualization, In Proceedings of the IEEE/ACM SC2002 Conference, Baltimore, Maryland, November, 2002.
J. Simpson, A.R. Sanderson, E. Luke, K. Balling, R. Coffey.
Collaborative Remote Visualization, In Presented before the Utah State Legislature, 2002.
J. Stinstra, M. Peters.
The Influence of Fetoabdominal Tissues on Fetal ECGs and MCGs, In Arch Physiol Biochem, Vol. 110, No. 3, pp. 165--176. 2002.
B. Taccardi, B.B. Punske, R.S. MacLeod, Q. Ni.
Visualization, Analysis and Physiological Interpretation of Three-dimentional Cardiac Electric Fields, In Proceedings of 24th Annual International Conference of the IEEE Engineering in Medicine and Biology Society, 2002.
T. Tasdizen, R.T. Whitaker, P. Burchard, S. Osher.
Geometric Surface Smoothing via Anisotropic Diffusion of Normals, In Proceeding of IEEE Visualization 2002, pp. 125--132. 2002.
X. Tricoche, T. Wischgoll, G. Scheuermann, H. Hagen.
Topology Tracking for the Visualization of Time-Dependent Two-Dimensional Flows, In Computer & Graphics, Vol. 26, pp. 249--257. 2002.
X. Tricoche, G. Scheuermann, H. Hagen.
Scaling the Topology of Symmetric Second Order Tensor Fields, In Hierarchical and Geometrical Methods in Scientific Visualization, Springer, Berlin, pp. 171-184. 2002.
X. Tricoche.
Vector and Tensor Topology Simplification, Tracking, and Visualization, Note: Schriftenreihe Fachbereich Informatik (3), Universitat Kaiserslautern, 2002.
R. Van Uitert, C.R. Johnson.
Can a Spherical Model Substitute for a Realistic Head Model in Forward and Inverse MEG Simulations?, In Proceedings of The 13th International Conference on Biomagnetism, Jena, Germany, August, 2002.
A.I. Veress, J.A. Weiss, G.T. Gullberg, D.G. Vince, R.D. Rabbitt.
Strain Measurement In Coronary Arteries Using Intravascular Ultrasound And Deformable Images, In ASME J. Biom. Eng., Vol. 124, pp. 734--741. 2002.
M. Walkley, P.K. Jimack, M. Berzins.
Anisotropic Adaptivity for Finite Element Solutions of 3-D Convection-Dominated Problems, In Int. J. Numer. Meth. Fluids, Vol. 40, No. 3-4, pp. 551--559. 2002.
M. Walkley, M. Berzins.
A finite element model for the two-dimensional extended Boussinesq equations, In International Journal for Numerical Methods in Fluids, Vol. 39, No. 10, pp. 865--885. 2002.
J.A. Weiss, J.C. Gardiner, C. Bonifasi-Lista.
Ligament Material Behavior is Nonlinear, Viscoelastic and Rate-Independent Under Shear Loading, In Journal of Biomechanics, Vol. 35, pp. 943--950. 2002.
R.T. Whitaker, V. Elangovan.
A Direct Approach to Estimating Surfaces in Tomographic Data, In J. Med. Img. Anal., Vol. 6, No. 3, pp. 235--249. 2002.
R.T. Whitaker, J. Gregor.
A Maximum Likelihood Surface Estimator for Dense Range Data, In IEEE Trans. Pattern Anal. & Mach. Intel., pp. 1372--1387. 2002.
R.T. Whitaker, E. L. Valdes-Juarez.
On the Reconstruction of Height Functions and Terrain Maps from Dense Range Data, In IEEE Trans. Imag. Proc., Vol. 11, No. 7, pp. 704--716. 2002.
D. Xiu, G.E. Karniadakis.
The Wiener-Askey Polynomial Chaos for Stochastic Differential Equations, In SIAM Journal on Scientific Computing, Vol. 24, No. 2, pp. 619--644. 2002.
DOI: 10.1137/S1064827501387826
We present a new method for solving stochastic differential equations based on Galerkin projections and extensions of Wiener's polynomial chaos. Specifically, we represent the stochastic processes with an optimum trial basis from the Askey family of orthogonal polynomials that reduces the dimensionality of the system and leads to exponential convergence of the error. Several continuous and discrete processes are treated, and numerical examples show substantial speed-up compared to Monte Carlo simulations for low dimensional stochastic inputs.
Keywords: polynomial chaos, Askey scheme, orthogonal polynomials, stochastic differential equations, spectral methods, Galerkin projection
D. Xiu, D. Lucor, C.-H. Su, G.E. Karniadakis.
Stochastic Modeling of Flow-Structure Interactions using Generalized Polynomial Chaos, In Journal of Fluids Engineering, Vol. 124, No. 1, pp. 51--59. 2002.
DOI: 10.1115/1.1436089
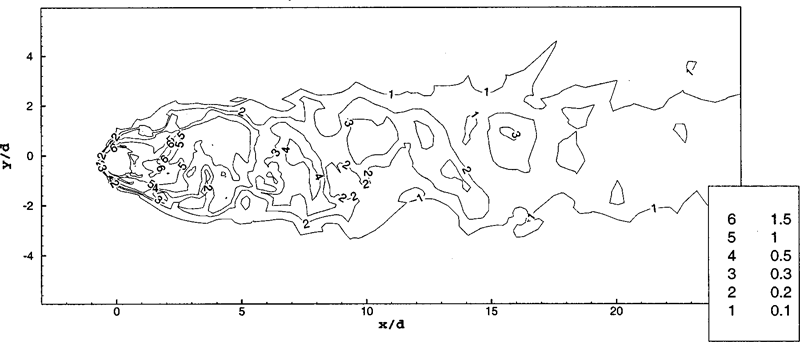
D. Xiu, G.E. Karniadakis.
Modeling Uncertainty in Steady State Diffusion Problems via Generalized Polynomial Chaos, In Computer Methods in Applied Mechanics and Engineering, Vol. 191, No. 43, pp. 4927--4948. 2002.
We present a generalized polynomial chaos algorithm for the solution of stochastic elliptic partial differential equations subject to uncertain inputs. In particular, we focus on the solution of the Poisson equation with random diffusivity, forcing and boundary conditions. The stochastic input and solution are represented spectrally by employing the orthogonal polynomial functionals from the Askey scheme, as a generalization of the original polynomial chaos idea of Wiener [Amer. J. Math. 60 (1938) 897]. A Galerkin projection in random space is applied to derive the equations in the weak form. The resulting set of deterministic equations for each random mode is solved iteratively by a block Gauss–Seidel iteration technique. Both discrete and continuous random distributions are considered, and convergence is verified in model problems and against Monte Carlo simulations.
Keywords: Uncertainty, Random diffusion, Polynomial chaos
Page 119 of 142